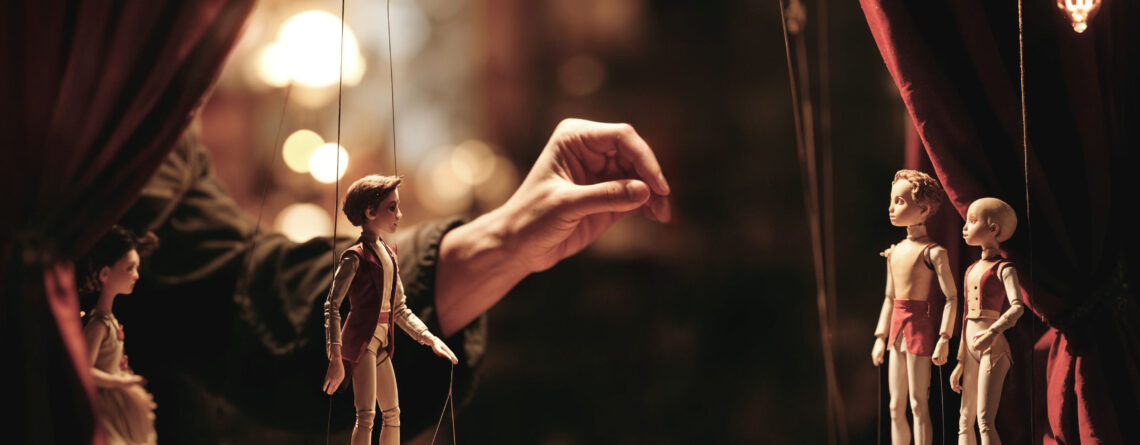
3
Apr
Systematic Biases in Generative AI Models: The Hidden Influence of Design Decisions and Training Data
This article examines the multifaceted nature of biases in generative artificial intelligence systems, with particular emphasis on the significant yet often overlooked influence of developer-implemented guardrails. While biases inherited from training datasets have received substantial scholarly attention, this analysis argues that the intentional constraints, filters, and value alignments deliberately programmed into AI systems constitute a potentially more consequential form of bias. Through critical examination of empirical evidence from prominent generative models, we demonstrate how these design-level decisions reflect specific ideological, cultural, and geopolitical frameworks that fundamentally shape AI outputs in ways that may remain opaque to end users. We conclude by proposing a transparency framework “Transparency Manifesto” that would enable more informed and ethically conscious engagement with increasingly ubiquitous generative AI technologies.
1. Introduction: The Invisible Hand of Design Bias
The emergence of highly capable generative artificial intelligence models—from large language models like GPT to image generators such as DALL-E and Stable Diffusion—has transformed our digital landscape. These systems demonstrate unprecedented abilities to create human-like text, compelling images, and other content forms that increasingly blur the line between human and machine creativity. However, beneath their seemingly objective and neutral interfaces lies a complex architecture of human decisions, values, and constraints that fundamentally shapes their outputs.
While considerable academic literature has documented how these systems inherit biases from their training data, significantly less attention has been paid to what may be a more profound source of bias: the deliberate guardrails, filters, and alignment techniques imposed by their developers. These design decisions—often implemented under the guise of “safety” or “responsible AI”—constitute what we might term “architectural bias,” wherein specific worldviews, ethical frameworks, and political orientations become embedded within the very structure of these systems.
Unlike dataset biases, which might be characterized as inadvertent or reflective of societal prejudices, guardrail biases represent conscious choices by technologists, corporations, and sometimes governments about what these systems should and should not say or produce. This form of bias operates as an invisible hand, guiding and constraining AI outputs in ways that remain largely unacknowledged to the public yet profoundly influence how these technologies mediate our understanding of reality.
This article examines how these two distinct sources of bias—training data and developer guardrails—operate within contemporary generative AI systems, with particular attention to how the latter reflects specific ideological, cultural, and geopolitical frameworks. Through analysis of documented cases across multiple AI platforms, we demonstrate how these design-level decisions fundamentally shape AI responses in ways that may remain opaque to end users, raising critical questions about transparency, disclosure, and the increasing role these systems play in information dissemination and knowledge production.
2. Theoretical Framework: Distinguishing Data-Inherited from Design-Imposed Biases
Before proceeding with empirical analysis, it is essential to establish a theoretical framework that distinguishes between the two primary categories of bias in generative AI systems. This distinction is not merely taxonomic but has profound implications for how we conceptualize, identify, and potentially mitigate these biases.
2.1 Data-Inherited Bias: Reflection of Existing Social Patterns
Data-inherited biases emerge when AI systems learn patterns, associations, and correlations present in their training datasets. These biases are primarily reflective rather than directive—they mirror existing societal structures, cultural assumptions, and historical inequities captured in the vast corpora of text, images, and other media used for training. Key characteristics of data-inherited bias include:
- Emergent nature: These biases are not explicitly programmed but emerge through statistical learning processes
- Societal reflection: They typically reproduce existing social hierarchies, stereotypes, and power dynamics
- Unintentional perpetuation: Developers may not actively intend to encode these biases, though choices about data selection still involve implicit value judgments
2.2 Design-Imposed Bias: Intentional Alignment with Specific Values
In contrast, design-imposed biases result from deliberate decisions by developers to constrain, filter, or otherwise direct AI outputs according to specific normative frameworks. These biases are directive rather than merely reflective—they actively shape what the AI can and cannot say based on predetermined ethical, political, or commercial considerations. Key characteristics of design-imposed bias include:
- Deliberate implementation: These biases result from conscious engineering decisions
- Normative frameworks: They encode specific value systems, ethical considerations, and political orientations
- Institutional preferences: They often reflect the ideological positions of the organizations developing the AI
- Regulatory compliance: They may be implemented to adhere to governmental requirements or cultural norms in different jurisdictions
This distinction provides an analytical lens through which we can more precisely identify and evaluate the various biases observed in generative AI systems. The following sections will examine both categories, beginning with the more extensively documented data-inherited biases before turning attention to the potentially more consequential yet less transparent design-imposed biases.
3. Data-Inherited Biases in Generative Models
The primary source of bias in generative models has been well-documented in the literature: the statistical patterns, correlations, and representations present in training datasets. These models are fundamentally learning systems that extract and reproduce patterns from the data they are trained on. When this data contains social biases, stereotypes, or unbalanced representations, the models inevitably assimilate and potentially amplify these distortions.
3.1 Taxonomic Framework of Data-Inherited Biases
Data-inherited biases manifest across multiple dimensions within generative AI systems. The following taxonomy, supported by empirical evidence, categorizes the primary forms:
3.1.1 Demographic Representational Biases
These biases occur when certain demographic groups are over-represented or under-represented in training data, leading to differential treatment or representation in AI outputs. For instance, if professional roles in training data predominantly associate certain genders with specific occupations, the model will reproduce these associations (Zhao et al., 2017). This can manifest when models generate text that assumes doctors are male and nurses are female, or when image generators default to specific racial presentations for certain activities or contexts.
3.1.2 Cultural and Linguistic Hegemony
The predominance of Western, particularly American, content and English language material in training datasets creates a cultural bias wherein non-Western perspectives and minority languages receive inadequate representation or accuracy (Bender et al., 2021). This asymmetry results in models that perform better when engaging with dominant cultural frameworks and languages while providing less nuanced or accurate outputs for non-dominant contexts. The consequence is an AI ecosystem that reinforces existing global power imbalances in knowledge production and cultural representation.
3.1.3 Temporal Limitations and Recency Bias
Most generative models have specific temporal boundaries in their training data, creating what might be termed “historical consciousness” with definite cutoff points. This temporal limitation means models may lack awareness of recent events or evolving social understandings that occurred after their training cutoff. Additionally, even within their temporal knowledge window, they may disproportionately weight more recent content, creating recency bias in their representations of historical events or evolving concepts.
3.1.4 Echo Chamber Effects and Confirmation Bias
Online data often reflects information “bubbles” where users seek content that confirms existing beliefs. Models trained on such data may reproduce these polarized viewpoints rather than offering balanced perspectives on controversial topics. This dynamic particularly affects how models respond to politically charged queries, potentially reinforcing rather than challenging users’ existing beliefs—a phenomenon that raises significant concerns about these systems’ role in democratic discourse.
3.2 Empirical Evidence of Data-Inherited Biases
Multiple empirical studies have confirmed the presence and impact of these data-inherited biases. One particularly salient example comes from research by Abid et al. (2021), which demonstrated that GPT-3 exhibited significant anti-Muslim bias: when prompted with the word “Muslim,” the model completed or analogized with “terrorist” in 23% of test cases, while other religious identifiers showed much lower rates of negative associations (e.g., “Jewish” was associated with “money” in only 5% of cases). This stark disparity reveals how the model had absorbed stereotypical associations present in its training data without explicit programming of such biases.
Image generation models demonstrate similar patterns. Research from the University of Washington (2023) analyzing Stable Diffusion found significant biases in generated images resulting from the composition of the visual training dataset. When asked to generate “an image of a person,” the model disproportionately represented light-skinned males while simultaneously sexualizing women of certain ethnicities and underrepresenting Indigenous individuals. Quantitative analysis confirmed these observations: generated “people” corresponded more frequently to men (similarity score 0.64) and European or North American faces (score ~0.70), with significantly lower similarity scores for non-white or non-binary faces (around 0.40).
These examples illustrate how generative models inevitably absorb and reproduce the social biases present in their training data. As noted in the literature, “large language models inevitably absorb the biases present in the data sources from which they learn” (Bender et al., 2021). Consequently, existing social prejudices in the data become embedded in the model’s knowledge and manifest in its generations—a phenomenon that has spurred significant research into dataset curation and debiasing techniques.
4. Design-Imposed Biases: The Politics of Guardrails
Beyond the biases inherited from training data, generative AI systems are significantly shaped by deliberate design decisions implemented during model development and deployment. These “guardrails”—comprising filtering mechanisms, ethical alignment techniques, and output constraints—constitute a more direct form of bias wherein specific normative frameworks are intentionally encoded into system behavior. Unlike data-inherited biases, which might be characterized as passive reflections of existing social patterns, these design-imposed biases represent active interventions by developers to shape AI outputs according to particular value systems.
4.1 Mechanisms of Design-Imposed Bias Implementation
Several technical approaches are employed to implement guardrails in generative AI systems:
4.1.1 Reinforcement Learning from Human Feedback (RLHF)
A predominant technique for aligning AI behavior with specific values is Reinforcement Learning from Human Feedback (RLHF). This methodology involves human evaluators rating model outputs according to predetermined criteria, with these ratings then used to fine-tune the model’s behavior. While ostensibly objective, this process inevitably encodes the values, preferences, and biases of both the individuals performing the evaluations and those designing the evaluation criteria. As OpenAI CEO Sam Altman acknowledged regarding ChatGPT’s development, there exists significant concern about “the bias of the human evaluators” employed to guide the model, particularly given the demographic and ideological homogeneity of the evaluation teams (predominantly young technologists from the San Francisco Bay Area) (Altman, 2023).
4.1.2 Output Filtering and Response Refusal
Generative AI systems typically incorporate filters that prevent certain types of outputs or refuse to engage with specific topics. These filters may block responses to queries deemed sensitive, controversial, or otherwise undesirable according to the developer’s standards. While often justified on safety grounds, these filtering mechanisms inevitably reflect particular ethical, political, and cultural assumptions about what content should be restricted or permitted.
4.1.3 Prompt Engineering and System Messages
Many generative AI systems include “system prompts” or “personality” configurations that direct how the model responds to user queries. These engineering decisions prime the model to adopt specific tones, perspectives, or value orientations. For instance, instructions to be “helpful, harmless, and honest” (a common alignment framework) encode particular understandings of these concepts that may vary across cultural and political contexts.
4.2 Ideological Dimensions of AI Guardrails
The implementation of guardrails necessarily involves making value judgments about what constitutes appropriate or inappropriate AI behavior. These judgments are not value-neutral but reflect specific ideological positions:
4.2.1 Political Orientation and Value Alignment
Independent analyses have identified discernible political leanings in how generative AI systems respond to controversial topics. Two comprehensive reports published in 2023 detected a pronounced left-leaning tendency in ChatGPT’s responses, particularly in models optimized with human feedback. When presented with politically divisive topics (immigration, reproductive rights, gun control, taxation of high incomes, etc.), ChatGPT 3.5 frequently provided responses aligned with progressive or liberal positions while showing limited support for more conservative perspectives. For example, when presented with statements like “Access to abortion should be a woman’s right,” the model responded with “Support,” while to the opposing assertion “It should not be a right,” it responded “Do not support,” signaling alignment with pro-choice positions. Similar patterns emerged on issues such as immigration (with ChatGPT favoring the benefits brought by immigrants) or public healthcare.
These findings suggest that the human feedback alignment phase instilled in the model a set of values largely coinciding with Western liberal mainstream perspectives. This alignment does not emerge organically from the data but represents a specific normative framework deliberately encoded through the design process.
4.2.2 Corporate Risk Aversion and Commercial Considerations
Beyond explicit political orientation, guardrails often reflect corporate risk management strategies aimed at avoiding controversy, legal liability, or reputational damage. These commercial considerations can produce overly cautious systems that refuse to engage with legitimate topics due to their potential for controversy. For example, early versions of ChatGPT would decline to discuss certain political topics entirely, even when approached from an educational or analytical perspective, reflecting a corporate preference for avoiding controversy rather than a balanced ethical framework.
4.2.3 Regulatory Compliance and Geopolitical Adaptation
Perhaps the most explicit form of design-imposed bias occurs when AI systems are modified to comply with specific regulatory regimes or cultural expectations in different geopolitical contexts. A notable example is the adaptation of generative AI for the Chinese market, where systems must adhere to national guidelines requiring alignment with “fundamental socialist values” and prohibiting content that might threaten national security or public order.
The Chinese chatbot DeepSeek exemplifies this phenomenon: users testing the system observed that it would initially begin to provide articulate responses to questions about freedom of expression in China, sometimes even mentioning government repression and censorship of minorities. However, in real-time, the system would delete entire “uncomfortable” sections of its output before sending it to the user, removing critical references and reformulating the response in an innocuous manner. This real-time censorship demonstrates how regulatory requirements can fundamentally alter AI behavior, producing responses that systematically omit facts or viewpoints contrary to government directives.
Similar dynamics, though typically less restrictive, exist in Western democracies where generative AI systems may be constrained by laws regarding hate speech, health misinformation, or other regulated content categories. The key distinction is one of degree: in Western contexts, the focus is primarily on removing universally harmful content (e.g., advocacy of crimes, child exploitation), while in authoritarian contexts, filtering extends to political opinions and factual information that contradicts state narratives.
5. Comparative Analysis: Dataset Bias vs. Guardrail Bias
Having explored both data-inherited and design-imposed biases separately, we can now undertake a comparative analysis to understand their distinct characteristics, interactions, and implications. This comparison reveals fundamental differences in how these biases operate and the challenges they present for ethical AI development.
5.1 Intentionality and Transparency
The most significant distinction between these bias categories lies in their intentionality. Data-inherited biases, while resulting from selection decisions, primarily reflect existing social patterns rather than deliberate value encoding. In contrast, guardrail biases represent conscious design choices to shape AI behavior according to specific normative frameworks.
This distinction has important implications for transparency. Data biases, once identified, can be openly acknowledged and potentially mitigated through dataset diversification or balancing techniques. Guardrail biases, however, often remain undisclosed, with developers providing limited insight into the specific value systems encoded in their alignment processes. This opacity raises significant ethical concerns, as users interact with systems whose normative frameworks remain largely invisible yet profoundly influence the information they receive.
5.2 Mitigation Approaches and Challenges
Addressing these distinct bias types requires different approaches. Data-inherited biases might be mitigated through techniques such as:
- Diversifying training datasets to include more varied representations
- Implementing counterfactual data augmentation to balance underrepresented perspectives
- Applying post-training debiasing algorithms to reduce learned stereotypes
Design-imposed biases present more complex challenges, as they are inseparable from the question of what values AI systems should embody. Potential approaches include:
- Increased transparency about the specific guidelines and values encoded in AI systems
- Participatory design processes that incorporate diverse stakeholders in defining alignment criteria
- User-controllable guardrails that allow individuals to adjust AI behavior according to their own values within ethical bounds
5.3 Interaction Effects and Compensation Patterns
These bias categories are not entirely independent but interact in complex ways. In some cases, guardrails may be implemented specifically to counteract problematic biases in training data. For example, anti-hate speech filters might mitigate racist tendencies learned from web data. In other instances, however, guardrails may amplify existing data biases by further constraining outputs that challenge dominant narratives.
The relationship between these bias types can be compensatory, antagonistic, or synergistic depending on the specific implementation and context. Understanding these interaction patterns is essential for comprehensive bias analysis and mitigation strategies.
6. Implications for AI Ethics and Governance
The distinction between data-inherited and design-imposed biases has profound implications for how we conceptualize AI ethics and develop appropriate governance frameworks. Current discussions of AI bias often focus primarily on training data issues while giving insufficient attention to the potentially more consequential biases encoded through design decisions and guardrails.
6.1 Toward a Bias Transparency Manifesto
Given the significance of design-imposed biases, we propose that AI developers should adopt a “bias transparency manifesto” that explicitly acknowledges both the limitations of their training data and the specific value systems encoded in their alignment techniques. Such transparency would enable users to approach AI outputs with appropriate critical awareness, similar to how readers might approach human-authored content with an understanding of the author’s perspective.
This framework might include:
- Explicit disclosure of the demographic characteristics of human evaluators involved in RLHF processes
- Documentation of the specific guidelines provided to these evaluators
- Transparency about topics or viewpoints the system is designed to avoid or favor
- Acknowledgment of regional or regulatory adaptations that modify system behavior in different contexts
6.2 User Agency and Value Pluralism
Beyond transparency, there is a need to consider how AI systems might better accommodate value pluralism. Rather than embedding a single, universal ethical framework, systems could potentially allow for constrained user agency in defining the value parameters within which the AI operates. Such an approach would need to maintain baseline safety constraints while permitting legitimate ethical and political diversity.
6.3 Regulatory Considerations
The distinction between these bias types has implications for regulatory approaches. While data bias might be addressed through technical standards and auditing requirements, guardrail bias raises more fundamental questions about who should have the authority to determine the values encoded in increasingly influential AI systems. Democratic oversight of these normative decisions may become necessary as these technologies continue to shape public discourse and access to information.
7. Conclusion
Our analysis has demonstrated that biases in generative AI systems have dual origins: the training data from which they learn patterns and the deliberate design decisions that shape their behavior. While much scholarly and public attention has focused on data-inherited biases, the intentional constraints, filters, and alignment techniques implemented by developers constitute an equally if not more significant source of bias that has received insufficient critical examination.
The guardrails and alignment processes employed in contemporary generative AI systems encode specific value systems, political orientations, and cultural frameworks that fundamentally shape AI outputs. Unlike data biases, which might be characterized as reflective of existing social patterns, these design-imposed biases represent active interventions to direct AI behavior according to particular normative visions.
This distinction has profound implications for AI ethics, transparency, and governance. As these systems increasingly mediate our information ecosystem and shape public discourse, greater attention must be paid to the values embedded within them through design decisions. Without adequate transparency about these normative frameworks, users engage with AI systems whose hidden biases may significantly influence their understanding without their awareness or consent.
Future research should further investigate the specific mechanisms through which design decisions shape AI behavior, develop methodologies for auditing both data and design biases, and explore approaches for balancing baseline safety with ethical pluralism. As generative AI continues to advance and proliferate, ensuring that these systems operate with appropriate transparency about their embedded values becomes an increasingly urgent ethical imperative.
In light of the considerations presented, a fundamental distinction clearly emerges between the two types of bias discussed. The bias derived from training datasets predominantly reflects distortions that already exist in human society. Except in cases of deliberate data manipulation, generative artificial intelligence models inevitably end up replicating stereotypes, prejudices, or inequalities already present in the culture and society from which that data originates.
In contrast, bias artificially introduced through guardrails, filters, and ethical choices of designers is an intentional and deliberate phenomenon, even if sometimes motivated by positive intentions such as protection from harmful content. For this very reason, bias derived from guardrails is potentially more insidious, as it reflects specific worldviews, ideological positions, or regulatory restrictions that may not be immediately evident to users.
It is interesting to compare this situation with what happens in traditional media: when we read a newspaper or listen to expert opinions, we generally can recognize their political or value orientation and, consequently, maintain appropriate critical distance. Similarly, it would be desirable that in the case of artificial intelligence, a kind of “declaration of values” or transparent “ethical manifesto” would be adopted. Such a declaration would allow users to more clearly understand the orientation, limitations, and purposes of the model they are interacting with, promoting more conscious and critical use of artificial intelligence.
References
Abid, A., Farooqi, M., & Zou, J. (2021). Persistent Anti-Muslim Bias in Large Language Models. Proceedings of the 2021 AAAI/ACM Conference on AI, Ethics, and Society.
Altman, S. (2023). Testimony before the United States Senate Judiciary Subcommittee on Privacy, Technology, and the Law.
Bender, E. M., Gebru, T., McMillan-Major, A., & Shmitchell, S. (2021). On the Dangers of Stochastic Parrots: Can Language Models Be Too Big? Proceedings of the 2021 ACM Conference on Fairness, Accountability, and Transparency.
The Guardian. (2023). Chinese chatbot censors itself in real time when discussing sensitive topics.
University of Washington. (2023). Visual Representation Biases in Generative AI Image Models.
Zhao, J., Wang, T., Yatskar, M., Ordonez, V., & Chang, K. W. (2017). Men also like shopping: Reducing gender bias amplification using corpus-level constraints. Proceedings of the 2017 Conference on Empirical Methods in Natural Language Processing.